R-loops are prevalent triple helices that play regulatory roles in gene expression and are involved in various diseases. Our work improves the understanding of the relationship between the nucleotide sequence and DNA topology in R-loop formation. We use a mathematical approach from formal language theory to define an R-loop language and a set of rules to model R-loops as words in that language. We train the resulting R-loop grammar on experimental data of co-transcriptional R-loops formed on different DNA plasmids of varying topology. The model accurately predicts R-loop formation and outperforms prior methods. The R-loop grammar distills the effect of topology versus sequence, thus advancing our understanding of R-loop structure and formation.
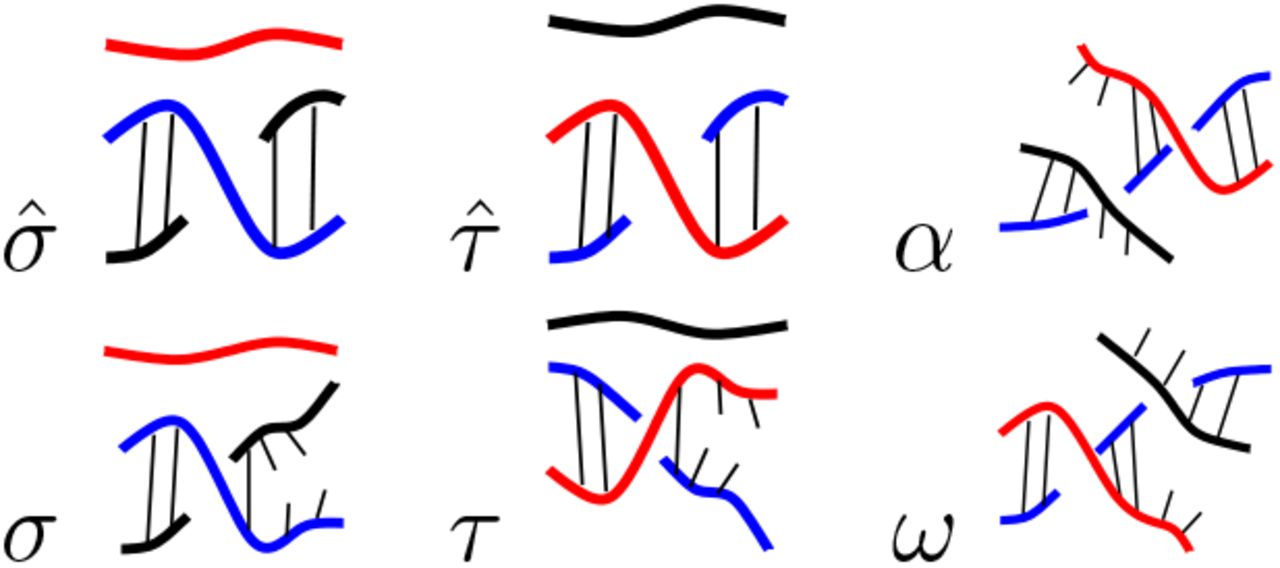